Edge AI: The Ultimate Guide to Vision Processing Units (VPUs)
It's Friday. I'm checking my phone for the 30th time in the last hour. It's 1:02 P.M. Something's wrong. The UPS courier is late. They said it would arrive by 1 P.M β why is it not here? I was preparing one of my fresh daily emails, but I couldn't focus. And suddenly... DING DONG! π He's here! I suddenly felt super excited. It felt like Christmas. This morning, I received my OAK-D Camera...
The OAK-D S2 is a stereo camera released by Luxonis, and it's a great device to use when doing Stereo Vision, Depth Estimation, 3D Reconstruction, and all these fancy 3D Computer vision applications. It is now part of many educational autonomous robots, like the Turtlebot 4 from Clearpath Robotics or the RAE from Luxonis. It also powers autonomous trucks, excavators, cars, robots, and tons of smart vision devices.
There is something special about this camera: its processor is what we call a VPU: A Vision Processing Unit.
You may have noticed it by now, Vision Processing Units are being integrated in many edge devices, whether it's cameras, or simply USB-Sticks.
But what are VPUs? How do they work? Why are they needed? How can you interface with them? And when should you use them? In this article, we're going to have an exploration of Vision Processing Units.
Let's begin:
What is a VPU, and what is the difference between VPUs and GPUs?
One of the most asked question in the Deep Learning world is: βWhich computer should I purchase?" And given that computers evolve very fast, this question gets asked every year. If you've asked the question yourself, you probably already heard the most common answer, which is to purchase a laptop that has an NVIDIA GPU.
A GPU (Graphical Processing Unit) is a chip designed to handle tasks related to graphics rendering and computation. Unlike a CPU that processes operations one by one, GPUs process operations in parallel.
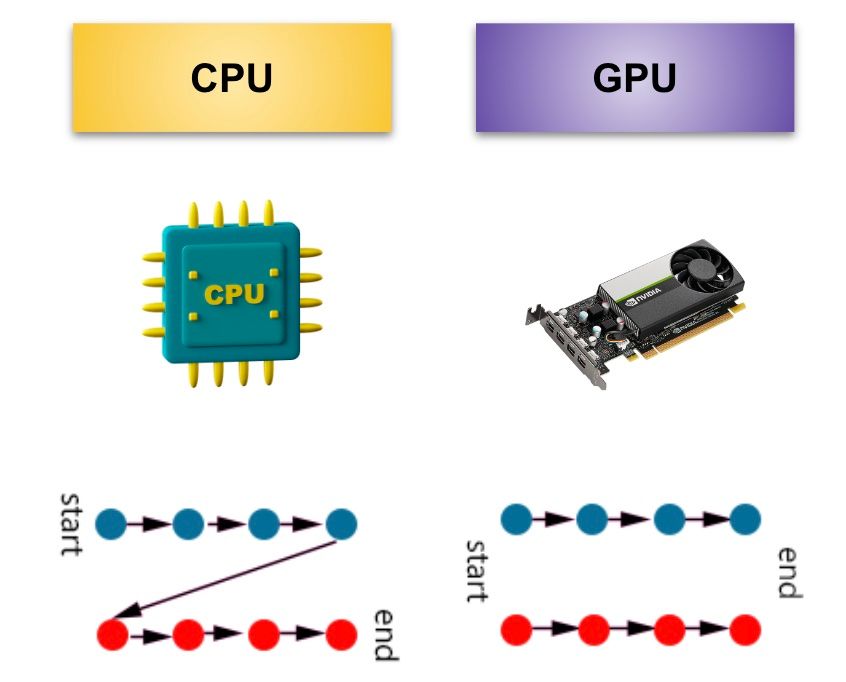
Why an "NVIDIA" GPU? By now, we know that Deep Learning requires lots of computation (hence GPUs), but if you purchase a GPU from NVIDIA, you also get something called CUDA and cuDNN, which as accelerators for your deep learning models that work with their GPUs.
But a GPU from NVIDIA is no longer the only "valid" solution. A few years ago, Intel released a new kind of chip that until now has been under the radar. A chip that is both very small and very powerful: A Vision Processing Unit (VPU).
Unlike a GPU, a VPU is specifically built for Machine Learning, and in particular for Computer Vision. Algorithms like Convolutional Neural Networks, image processing, or other uses of OpenCV or other libraries, are by default faster when using the VPU chip.
When looking at the full spectrum, you can see 5 types of chips:
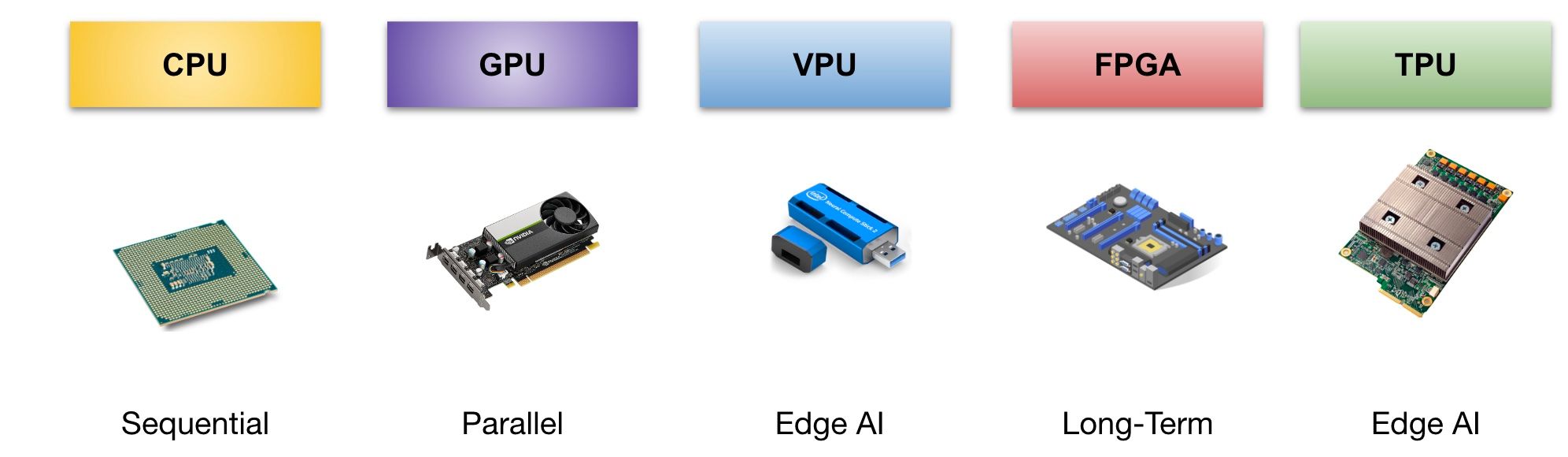
- CPUs (Central Processing Units)
- GPUs (Graphical Processing Units)
- iGPUs (integrated GPUs on the CPU)
- FPGAs (Field Programmable Gate Arrays)
- TPUs (Tensor Processing Units)
- VPUs (Vision Processing Units)
You already know the difference between CPUs and GPUs (and iGPUs). What about the others?
Field-Programmable Gate Arrays (FPGAs) are chips designed with maximum flexibility, so that they can be reprogrammed as needed in the field (even after manufacturing and deployment). They are also super robust, performant, quite costly (can go up to several thousand $), and they have a long lifespan (10 years).
TPUs and VPUs work differently. It's not that they are more or less powerful, but unlike the others, VPUs have been designed specifically for Computer Vision and Neural Networks. They're small, low cost (100-200$), and also ready-to-deploy.
So we know that VPUs are fast, and they're optimized for Neural Networks, Deep Learning, Computer Vision, and Deployment.
But what does a VPU look like?
Inside a Vision Processing Unit
In 2017, Intel announced the release of a revolution in Deep Learning: the Neural Stick Compute 2.

It's a USB Stick that can be plugged to any computer to give it power. When you plug the USB stick to your computer, you can transfer the computations to the VPU chip inside, and thus have a dedicated place for your machine vision algorithms. It keeps your computer completely free to do different operations.
How much power does this USB stick give? The power of its chip: the Movidius Myriad X.
The Movidius Myriad X
Let's begin with the tech: here's the high-level architecture of this chip.
What do you notice?

If we list down the elements of the chip, you can see may of them are talking about "Neural", "Images", etc... It reinforces the idea that VPUs are for Computer Vision applications.
Some Details:
- The Interface unit is the part that interacts with the host (computer, drone, etc...)
- The Imaging accelerators have specific kernels that are used for image processing operations such as denoising, edge detection, etc...
- The Neural compute engine is a dedicated hardware accelerator optimized for running deep neural networks at low power without any loss in accuracy (so imaging accelerator is for computer vision, and neural engine is for deep learning).
- Vector processors can break up a complex instruction and then execute many tasks in parallel, similar to a GPU.
- 2 On-chip CPUs are part of The Myriad X VPU: one is used to run the interface unit, and the other is used for on-chip coordination between the 3 other components.
Where are VPUs used?
VPUs aren't limited to USB sticks. In fact, the chip inside the stick, called the Movidius Myriad X, is also the same chip inside the OAK-D Camera I received. With that, the USB stick is no longer distributed. Today, devices get released directly with a VPU chip.
It is however a good tool for AI acceleration, for example when plugged to a drone:
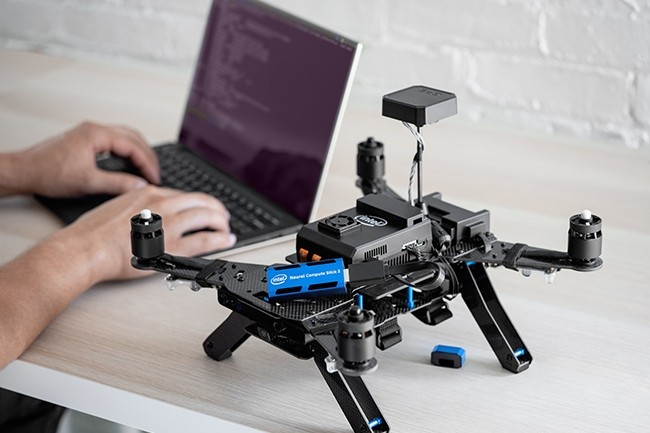
When using this chip, devices like the OAK-D camera can run any model (even custom models) at 4 TOPS of processing power, which is a lot.
OAK-D Demo
Back in 2017, Intel released a video showing their chip doing pedestrian detection, age estimation, gender classification, face detection, body orientation, and mood estimation; all at over 120 FPS. These are 6 different neural networks running on a tiny device.
In summer 2022, I started experimenting with Deep Stereo Vision for my OAK-D Playbook, and one of the applications was to combine Image Segmentation with Depth Estimation β and notice how these Neural Nets run at 26 FPS, on the camera's VPU chip alone.
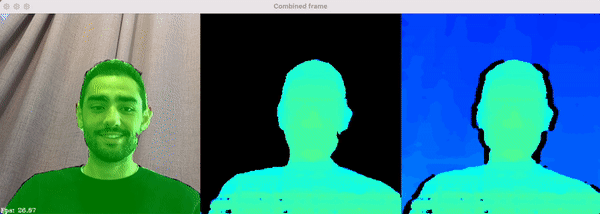
So this is all really fast. But how? Let's say you have a Neural Network, trained on Tensorflow, how do you make that super fast and ready to deploy?
Two words:
Open VINO.
Open VINO and how VPU makes magic β₯οΈ
Let's get concrete. You do you make a model 5 or 10 times faster with the VPU chip? Let's say you have a model trained on Keras, how do you export this to a NCS2 stick? or a VPU-powered camera like the OAK-D?
It's using toolkits like OpenVINO.
OpenVINO is the toolkit that communicates between a chip (like a VPU) and the other AI libraries.
Here is an example of how it interfaces between an algorithm written in Tensorflow and the VPU chip.
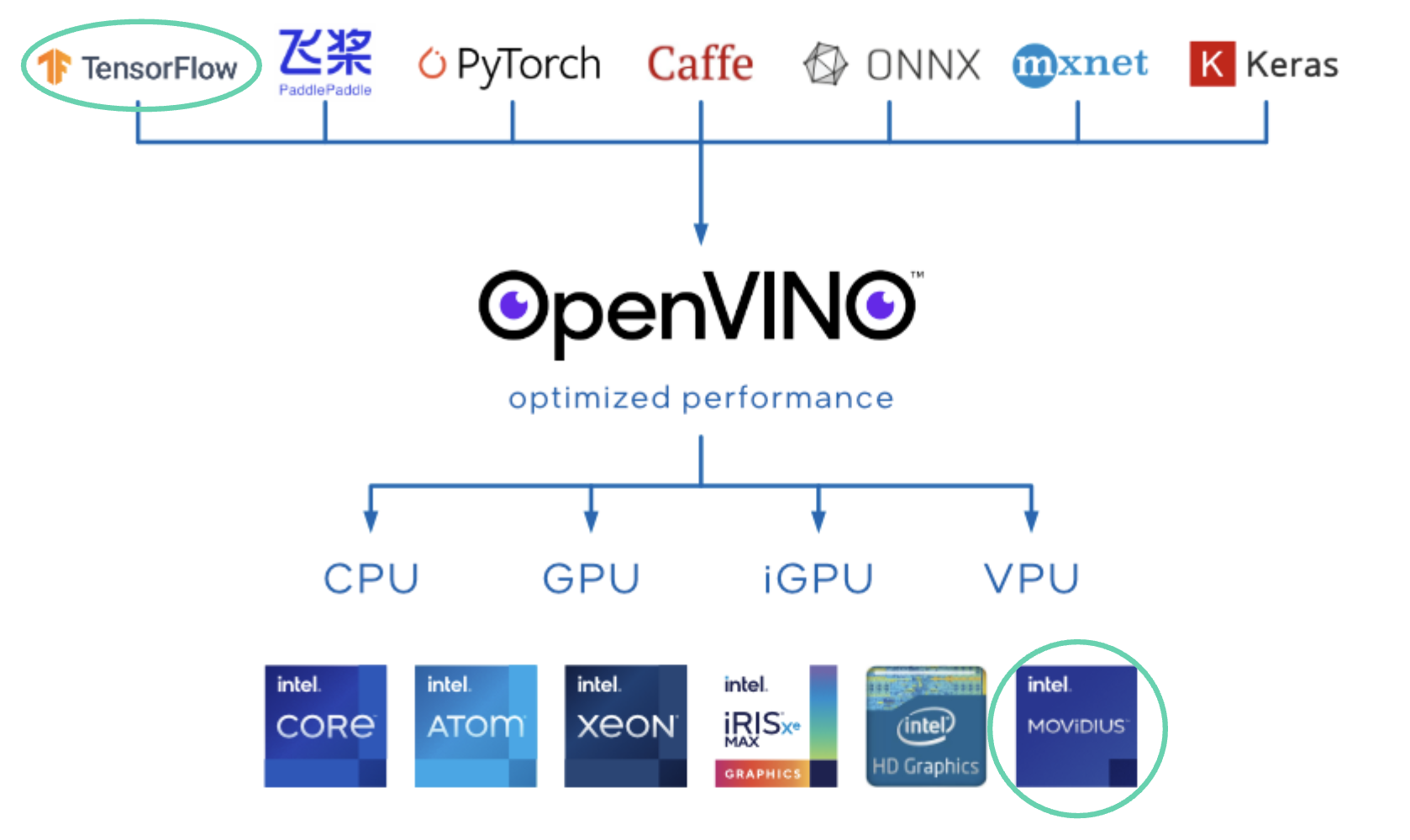
It really is the middle man. You have many algorithms written in any framework like PyTorch, Keras, Caffe, Tensorflow, and OpenVINO is going to export them to the VPU chip.
It does so according to the following drawing:
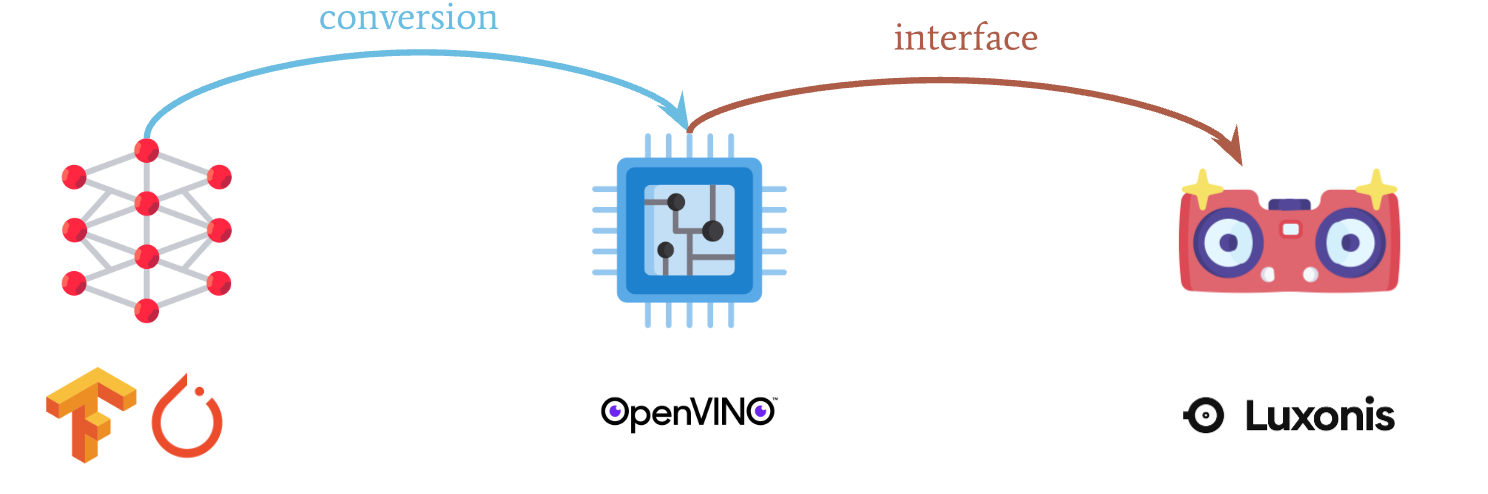
When we were running "normal" algorithms, we didn't care much about the VPU optimization. We wanted to optimize them in general, to make them faster, but now we have that extra step of optimising them specifically for the VPU chip.
Notice how it's a 2 step process:
- Conversion
- Interface
Conversion is about exporting your model to be OpenVINO compatible. For example, if you want your model to interface with the chip, you would act here. Interface is about communicating between the hardware (the camera) and the OpenVINO Library. For example, if you want to access the right camera of a Stereo Vision camera, you would act here.
Conversion: How to make your models "VPU compatible"
Here is what Intel shared when releasing OpenVINO.
To comment it:
- Get Your Model: A model is trained with Tensorflow or any other library
- Run Model Optimizer: It's then optimized using techniques such as quantization or pruning, explained in detail in my Neural Optimization course
- IR: Then, it's converted to Intermediate Representation (a .xml or .bin file detailing your network in an OpenVINO compatible way)
- OpenVINO Runtime: It's then sent to the inference engine where it can run.
- User Application: We're talking here about the interface, which is our next point.
So we know that you need to convert a model to OpenVINO format to make it compatible with a VPU. The process is explained on Intel's website, and you can even drag and drop a model in some of their pages like this one. Any model, even custom models, can be converted to OpenVINO.
This same tool (blobconverter) can be used in command lines: `
pip install blobconverter
model= blobconverter.from_zoo(name="age-gender-recognition-retail-0013", shaves=6)
model_path = blobconverter.from_zoo(name="yolop_320x320",
zoo_type="depthai",
shaves=6)
age_gender_nn.setBlobPath(model)
These 4 lines of code translate a model that does age and gender recognition (multi-task learning) to OpenVINO.
Now, let's see the Interface with the camera.
Interface: How the chip communicates with the camera
The VPU is a chip that can run neural networks. But if you're using specific hardware, you need to write somewhere in your code how to interface between a camera, drone, robot, and the chip.
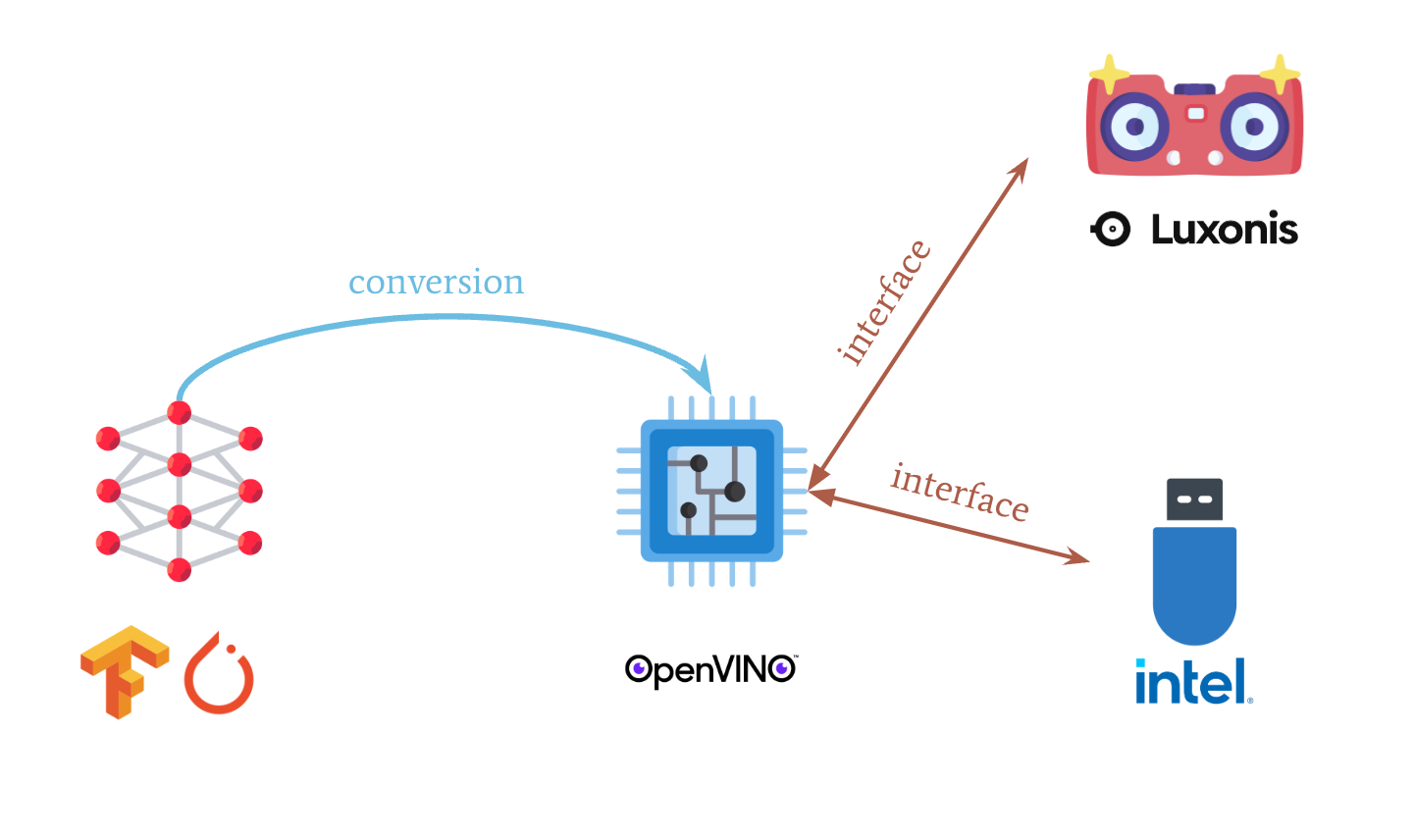
So, let's look at some code to understand better:
import depthai as dai
pipeline = dai.Pipeline()
nn = pipeline.create(dai.node.NeuralNetwork)
nn.setOpenVINOVersion(dai.OpenVINO.VERSION_2021_4)
nn.setBlobPath(str(Path("models/efficientdet_lite0_2021.3_6shaves.blob").resolve().absolute()))
with depthai.Device(pipeline) as device:
# Print Myriad X Id (MxID), USB speed, and available cameras on the device
print('MxId:',device.getDeviceInfo().getMxId())
print('USB speed:',device.getUsbSpeed())
print('Connected cameras:',device.getConnectedCameras())
A question, what do you think this code is doing?
You can clearly see 2 blocks:
- The first block is importing a library called Depth AI, and then defining objects, neural networks paths, and the OpenVINO version we'll use.
- The second block is connecting to the VPU chip, and printing its information such as the speed of the chip, the number of cameras, etc...
Yes, you may need a library like the DepthAI Library, or the OpenVINO Toolkit, that makes things easier, but you more than all needs to interface with the device. You need to tell which camera image you want to send to the neural network, or which USB stick you want to use, and on which port of your computer it's plugged.
When to use VPUs, and when not to
Vision Processing Units have several advantages, but it also has drawbacks to us them. Let's see when to use and not to use a VPU.
When to use a Vision Processing Unit
- It's all on the edge ; there is no interaction with the cloud. That means no latency and more privacy.
- It's also independent: you don't need to tweak your computer, you can allocate the processing power to the VPU and free the rest of your computer for other operations such as point cloud processing.
- It's affordable ~ 100-200$ for a device running this chip.
- It's versatile: you can use this chip to increase the speed of a Raspberry Pi, of an OAK-D camera, or simply your computer.
- It comes with a toolkit and SDK called OpenVINO that can implement deep learning CNN libraries on the dedicated Neural Compute Engine in TensorFlow and Caffe.
- Bonus: You can stack multiple USB sticks and double the power as long as you want.

When not to use a Vision Processing Unit
It's important to understand that a Vision Processing Unit is not a replacement for a GPU. It's an enhancement for CPUs when you want to use Machine Learning. If you have a Raspberry Pi and want to give it more power, you're going to use a VPU stick, or a Google Coral (edge TPU). If you have an autonomous robot, you may want to use a GPU for the main processing, and a VPU just for the camera.
For many scenarios, GPUs are still the go-to solution. In fact, if you have a high budget, and you have space (a GPU takes space), then it might be a better solution to go with a GPU. To learn more about how to deploy a project on the right hardware, you can read this article.
Conclusion and Summary
Let's go back to what we've looked at in this article:
- A Vision Processing Unit or VPU is a chip dedicated to Computer Vision and Machine Learning.
- Unlike a GPU, a VPU is affordable, small in size, and specifically built for Edge AI applications.
- The inside of a VPU device is a VPU chip, and the most common one is the Intel Movidius Myriad X.
- Today, embedded devices like the OAK-D Cameras, but also Raspberry Pi and drones are the perfect use case for VPUs.
- To make a model work with an Intel VPU, you need to use the OpenVINO toolkit to convert the models to an intermediate representation the chip understands, and then interface with the chip and camera.
- Keep in mind that VPUs = Embedded β In most cases, you want to pay attention to the details of a project before jumping on a processing solution.
- VPUs, although performant, don't have the same processing power as GPUs or FPGAs.
We are reaching an exciting era. VPUs have been implemented in drones, cameras, small robots, and a lot of IoT applications so far. It's still very early, but I expect the "edge" market to grow a lot in the coming years.
Imagine the possibilities when neither memory nor processing power is a significant obstacle. Every deep learning application we love, such as medicine, robotics, or drones, now can see the limitations drastically diminish.