Exactly how the Hungarian Algorithm works
If you're here, it's because you want to understand better the Hungarian Algorithm (or Kuhn Munkres algorithm)... This algorithm has saved me a lot of time when I was working on autonomous shuttles, and in particular on Multi-Object Tracking and Sensor Fusion. And in this article, I'll show you exactly how to apply it to almost any situation!
To kick off this article, I'd like to talk about 3 examples, and ask you to pay attention to the common element in these 3 scenarios:
1) You're getting married.
200 guests, champaign, bow tie, dresses, it's all going to be beautiful.
But a few days before the wedding, you remember that you forgot to plan the tables!
Where are your guests going to seat? And most of all, with who?
Your father is okay to sit with his in-law, but not with his brother! Your in-law is okay to sit with your parents, but not with their friends... And your two best friends who broke up last month? Should they sit together?
So this is scenario 1, and it takes us to scenario 2.
2) You're a Computer Vision engineer designing an object tracking algorithm for road surveillance.
For that, you decide to use a Multi-Object Tracking algorithm called SORT (you can learn more about it here). So you start with object detection, and in two consecutive frames, you can detect the yellow taxi in the picture!
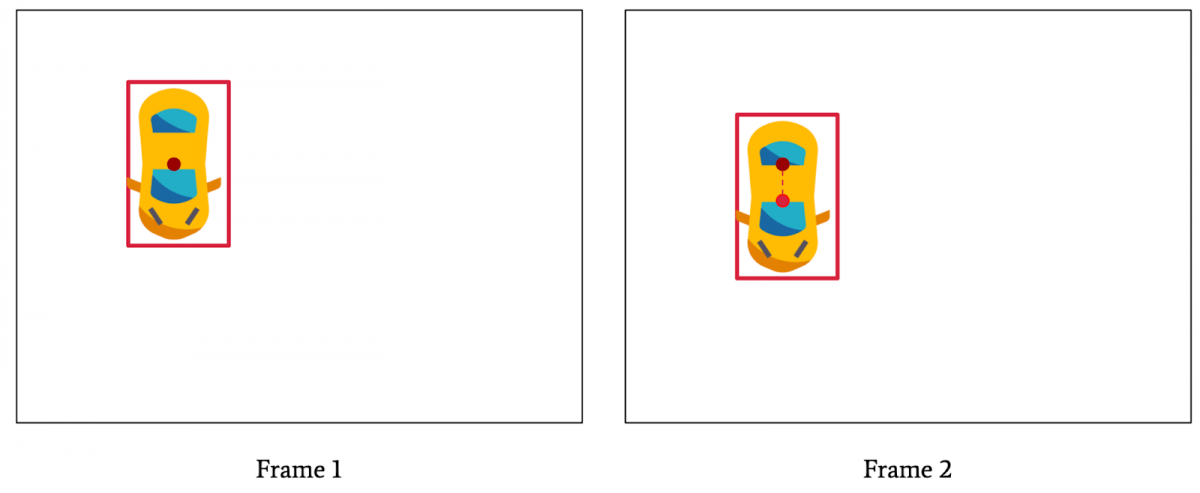
But suddenly, comes a second object, and it messes everything up!
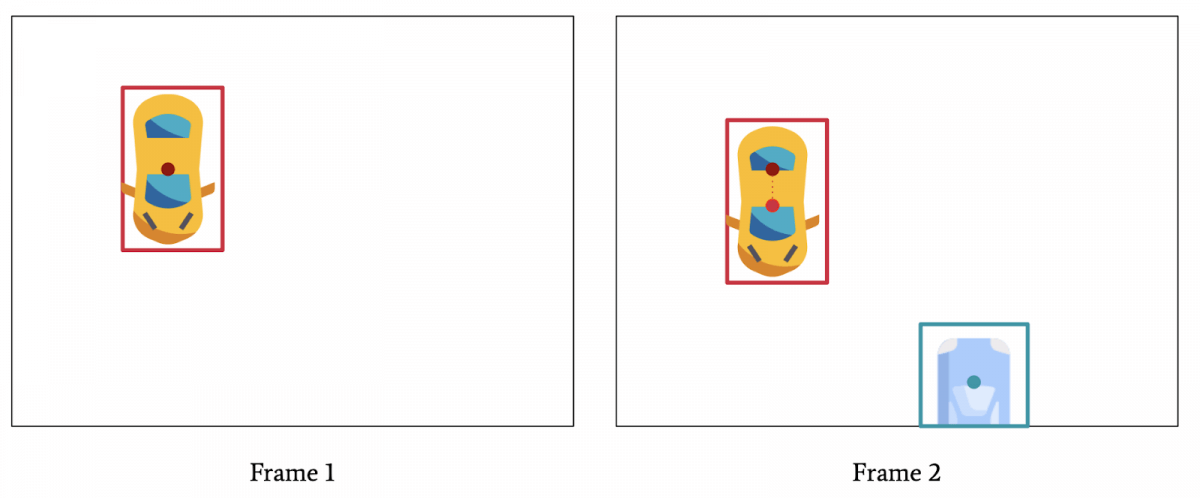
Now, we need to make sure the yellow cab has a red bounding box, and that this red bounding box isn't assigned to the new blue car. And what if 20 more cars show up? What if some of them are occluded? What if some disappear between frames? How do we assign the right IDs to the right objects?
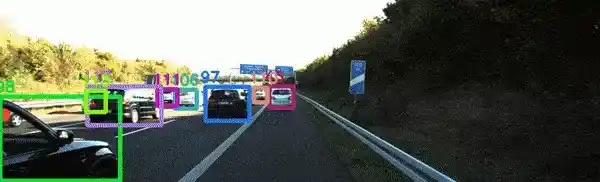
This takes us to scenario 3:
3) You're designing the Uber Algorithm to match drivers and clients.
Your job is to match drivers and clients based on their distances to each other. Every time a client orders a ride, the nearest driver is assigned the task, and drives to the client.
Now, what if there are 20 drivers near the client's location? What if some of them refuse rides below 20$? And what if there are 20 other clients waiting? What if some of them have a bad ranking? Or what if multiple of them have 5 stars?
How do you match the right clients to the right drivers so that everybody waits as little as possible?
Are you getting it?
These 3 examples all have one thing in common: they all solve an optimal assignment problem.
What is an optimal assignment problem?
Not all drivers can have the perfect and shortest ride, not all guests can seat with people they 100% approve... and not every bounding box can be perfectly tracked. But we still need to pair the elements, the best way we can.
In many fields, engineers use this algorithm, and today, we'll see how to use it in Self-Driving Cars! Yes, autonomous cars use this algorithm to pair obstacles from frame to frame, or to pair obstacles from sensor to sensor, as I describe in my 2 articles on Multi-Object Tracking and LiDAR and Camera Fusion. More, they also use it to pair 3D Tracks, as I describe in my 3D Object Tracking article.
To be sure you got the problem; here's a bonus example with this picture of a LiDAR camera fusion system, where the LiDAR has too many detections, and the camera misses detections. Again, we'll want to do a good assignment, and fuse the boxes... but based on what?
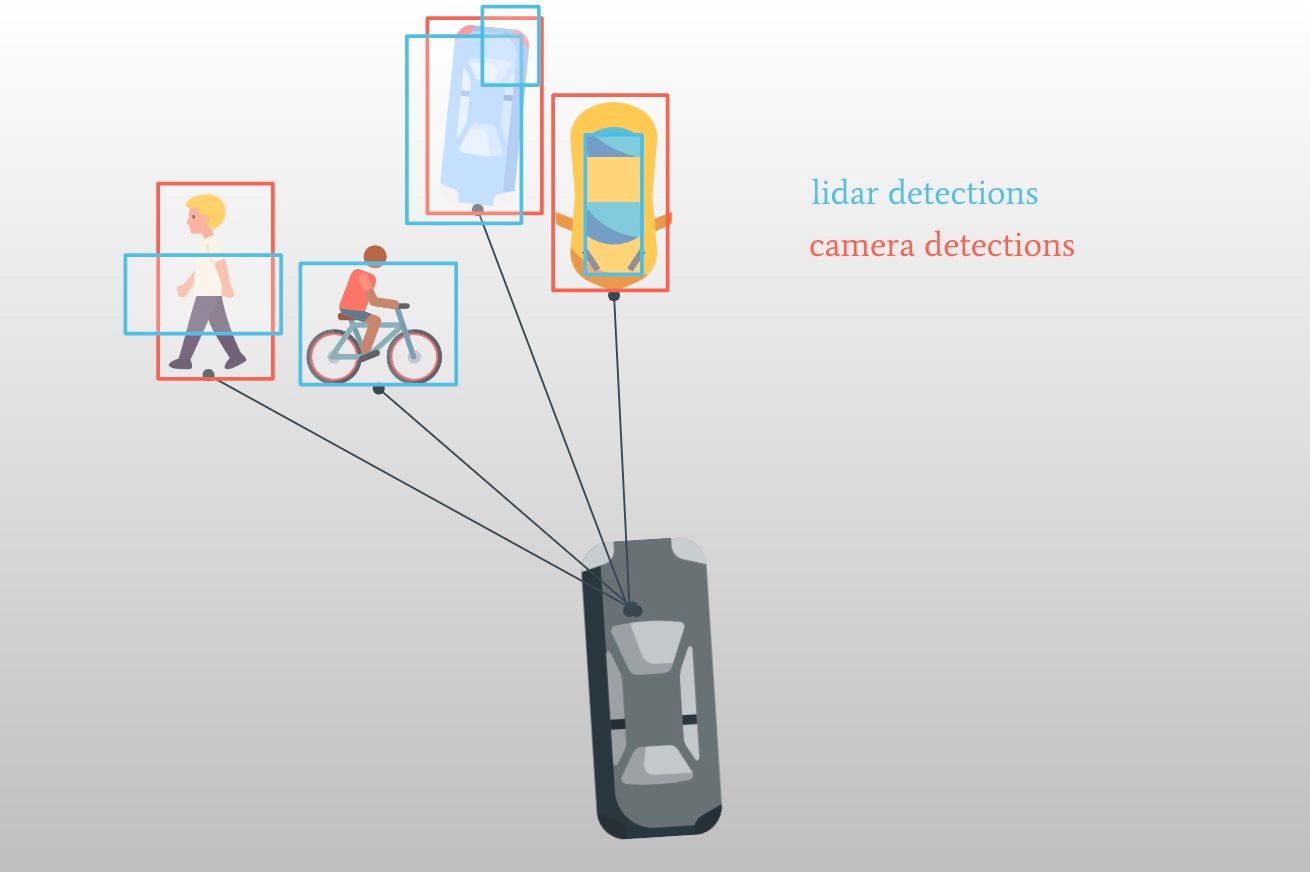
How the Hungarian Algorithm works
The example above shows exactly what we need to do: pair the blue boxes with the red boxes... but based on which criteria? The euclidean distance of the centers of the boxes? The IOU (Intersection Over Union) of the boxes, which represents an overlap? The visual similarity of the boxes? We have many options!
Let's begin with a definition...
What is Bipartite Graph Matching, and what does it have to do with the Hungarian Algorithm?
The Hungarian Algorithm is also named differently: Bipartite Graph Matching. The idea of Bipartite Graph Matching is to build a graph with distances, and to assign nodes from one side of the graph to the other.
If we take our fusion example, fusing LiDAR and Camera objects together, we could "look inside" every bounding box, and assign a score based on how similar the objects look. How much does the car detected in frame 1 look like the obstacles in frame 2? We set a score for each, and vote!
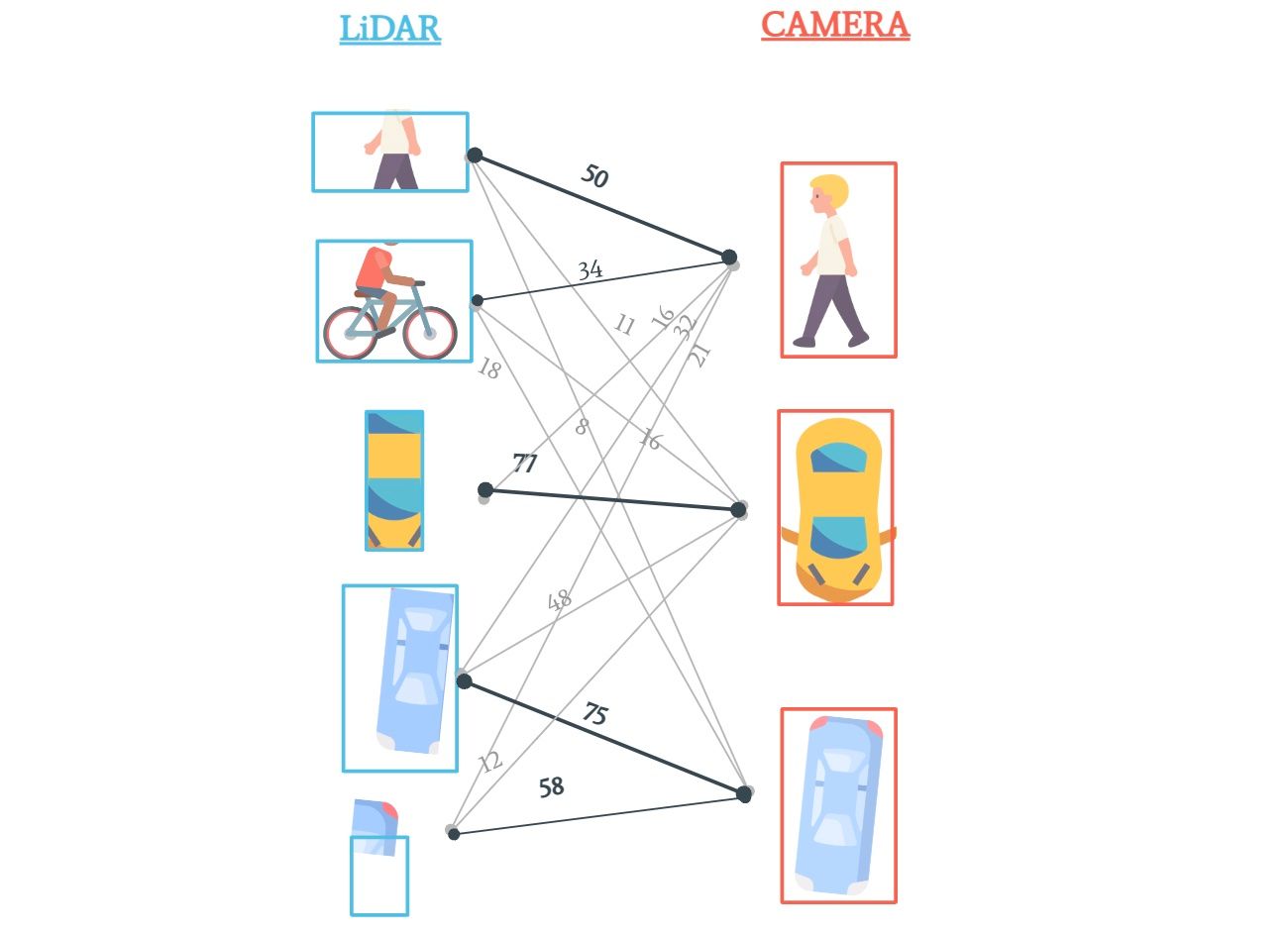
You can notice several issues here; such as the fact that some boxes can be matched with 2 elements, or that some boxes have no match!
This example is a bit hard, so instead, let's see a simpler one, and come back to this one right after.
Job vs Workers Example — Solving the Optimal Assignment Problem
To explain how the Hungarian Algorithm works, and is the best to use, I'll take an assignment from another example: Assigning Jobs to Workers based on the distance to the office.
Imagine you're living in a pre-COVID era, and remote work doesn't exist. You're in a company who's regularly sending employees to their clients houses to estimate their resell values. In this example, each house to estimate is considered a "job".
So how can we send real estates agent to the houses based on the shortest distance? We'll begin by putting everything in a matrix, 3 real estate agents, and 3 houses to estimate!
So here's a Matrix that translates our example.
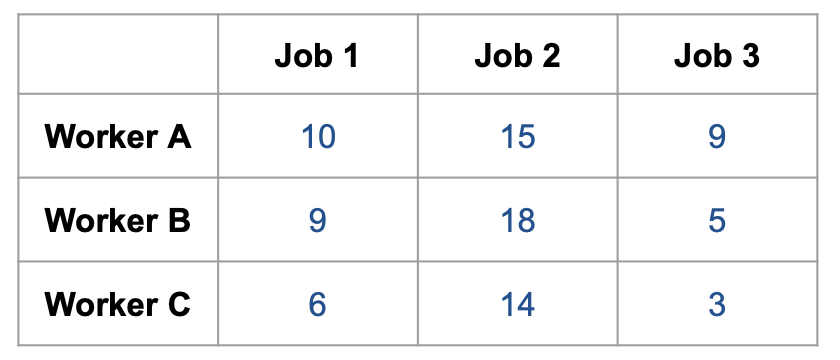
Pop Quiz — Intuitively, which job would you assign to which worker? It seems like all workers should go to job 3! So who should be satisfied?
The 5 Steps of the Hungarian Algorithm
We're not going to do anything brute force. Instead, we'll use a very methodical algorithm. Starting with a graph g, that becomes our original cost matrix that has n lines and n columns (n = 3 here), here's the pipeline:
- Subtract the minimum from every element of each row (this will make the smallest entry in the row now equal to 0).
- Subtract the minimum from every element of each column (this will make the smallest entry in the row now equal to 0).
- Cross the 0s with the minimum number of lines needed. If the number of lines is less than n (n=3), continue to step 4; otherwise, the optimal number of zeros has been reached and we can start pairing (go to step 5).
- Find the smallest entry not covered by any line, and subtract this entry to the entire matrix. If an element has been covered by any line twice, add it to the place where it’s double crossed. Then, go back to Step 3.
- Assign Jobs to Workers starting with the line with only one zero! Every time we're matching one job with a worker, we cross its row and column to make it unavailable.
I know, it looks completely crazy. In the following video, I apply the 5 steps to solve the problem.
Here’s a short recap of what we’ve done: Let’s apply the specific steps to our Job vs Worker example.
Step 1 — Subtract the minimum from every element of each row
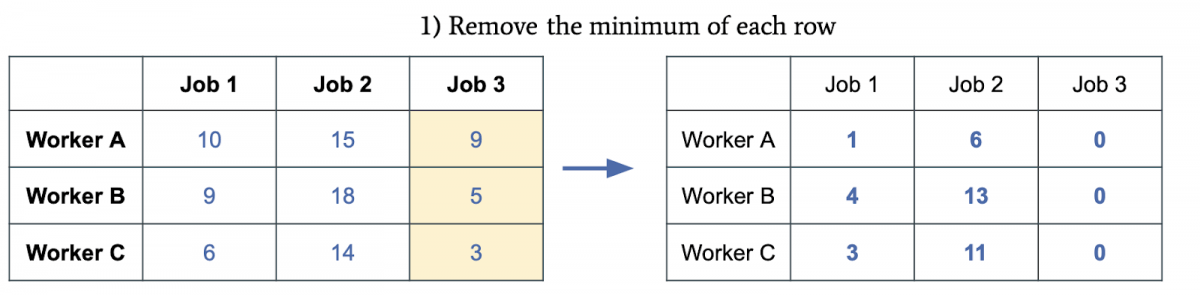
In our original matrix on the left, we can see that 9, 5, and 3 are the numbers to remove. We’re going to subtract 9 from the first row, 5 from the second row, and 3 from the third row.
Step 2 — Subtract the minimum from every element of each column
Second, we’ll do a similar job on the columns.
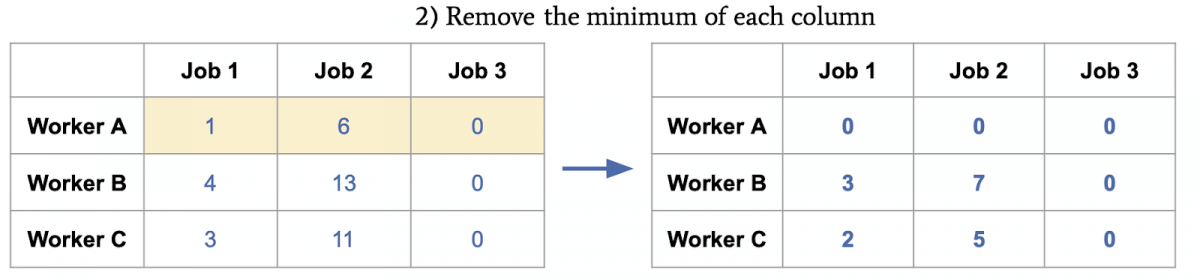
In our new matrix, we’ll subtract 1 from column 1, 6 from column 2, and 0 from column 3.
Step 3 — Cross 0s with the minimum number of lines needed.
This third step can be a bit tricky to understand. We want to cross 0s with the minimum number of lines we can do. Every time you see a 0, draw a line on either the row or the column.
In the following example, the left crossing is wrong, because it requires 5 lines to cover all 0s while we could do it with only 2 lines, as on the right example.

If you’re a developer, you probably start to think about how you would code this? Any ideas in mind?
The third step has a second part “then check the number of lines needed”. If that number is < n, the number of rows and columns in the matrix, reduce the matrix again.
In our example, we have 2 lines, but we have a 3x3 matrix, so we’ll reduce again. If we didn’t have this case, we’d directly jump to step 5.
Step 4 — Find the smallest entry not covered by any line, and subtract this entry to the entire matrix.
Okay, the inventor of the algorithm had to be drunk to get that step, or any other step.
Let’s take a look at the matrix now and see how we subtract 2 to the entire matrix, and then add it to the top right element, crossed twice..
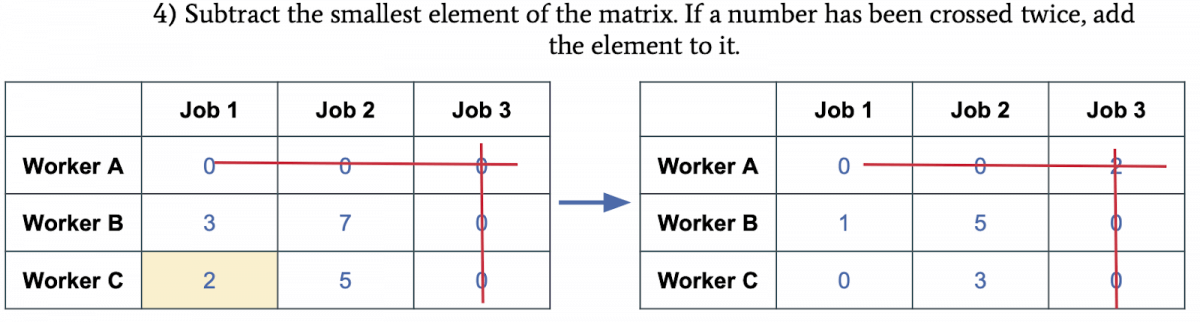
You’re probably wondering what number 5 is? Well, we’re almost done! Right before that, we’ll go back to step 3: cross the 0s!
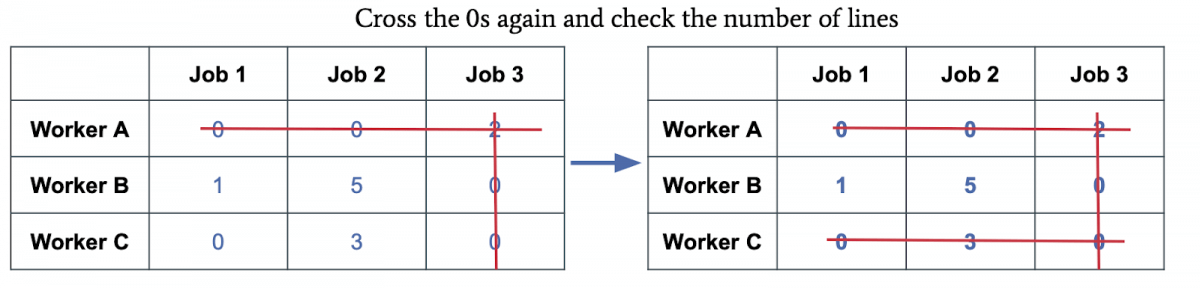
And at this moment, we have 3 lines, for 3 rows, so we pass the condition to pair workers and jobs!
Step 5 — Assign Jobs and Workers starting with the line with only one zero!
Every time we assign a job with a worker, cross its row and column to make it unavailable.
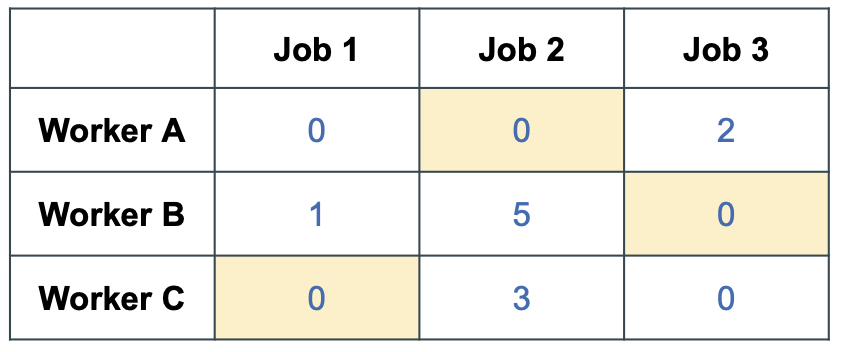
In the example, we begin with Worker B, as this is the only row with one zero. And if you’re wondering, yes, there will always be only one line with one zero, because we’ve reduced it to make it this way!
Now, we have what we call an optimal assignment! This is the best way to make everybody happy!
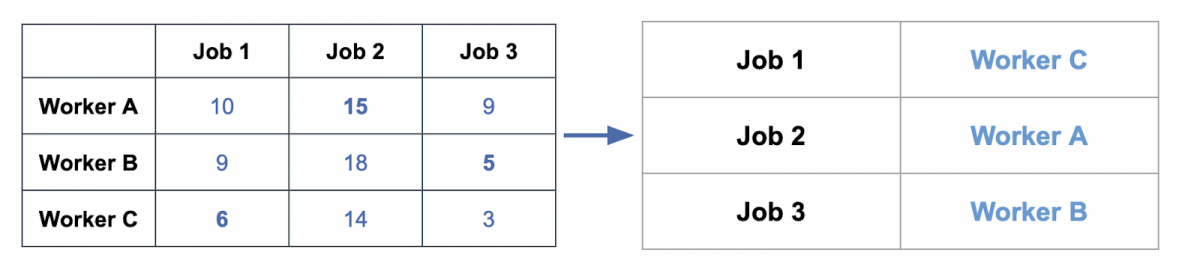
As you’ve noticed, we had to follow the algorithm scrupulously. And it worked because we had a perfect nxn matrix.
Many times, you won't have a perfect problem. You may want to pair 7 LiDAR boxes with 3 camera boxes, you may have noise and want to delete some boxes, you may want to solve a maximization problem rather than minimization.
How do we deal with this? This will be our next part!
Filling the Cost Matrix, and Solving Imperfect Problems
Before I talk about the cost matrix, I'd like to show you some of the things you can use when tracking bounding boxes. When pairing job and workers is an easy problem, pairing bounding boxes can be a very hard one.
So let's see what to do with it:
Possible Costs when doing bounding box assignment
Let’s take a look at a few possible costs when dealing with bounding box matching:
Euclidean Distance
First, we could assign bounding boxes to their closest centers by calculating the euclidean distance. Something like: d = √[(x2 – x1)2 + (y2 – y1)2]
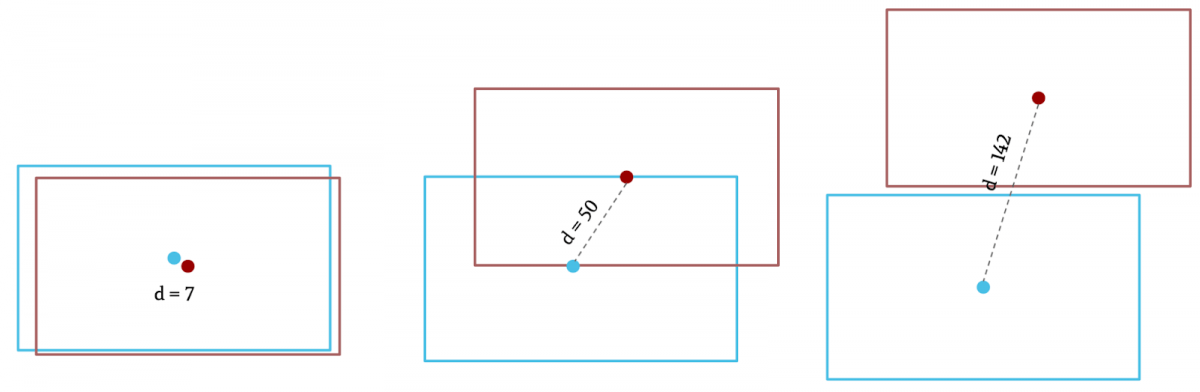
Although it would solve the problem for simple use cases, it certainly can't deal with issues such as overlapping obstacles, or changing shapes.
Intersection Over Union (IOU)
In the second suggestion, we could apply the Intersection Over Union. It’s a metric used to calculate how much overlap there are between two bounding boxes. Therefore, a cyclist wouldn’t match a car!
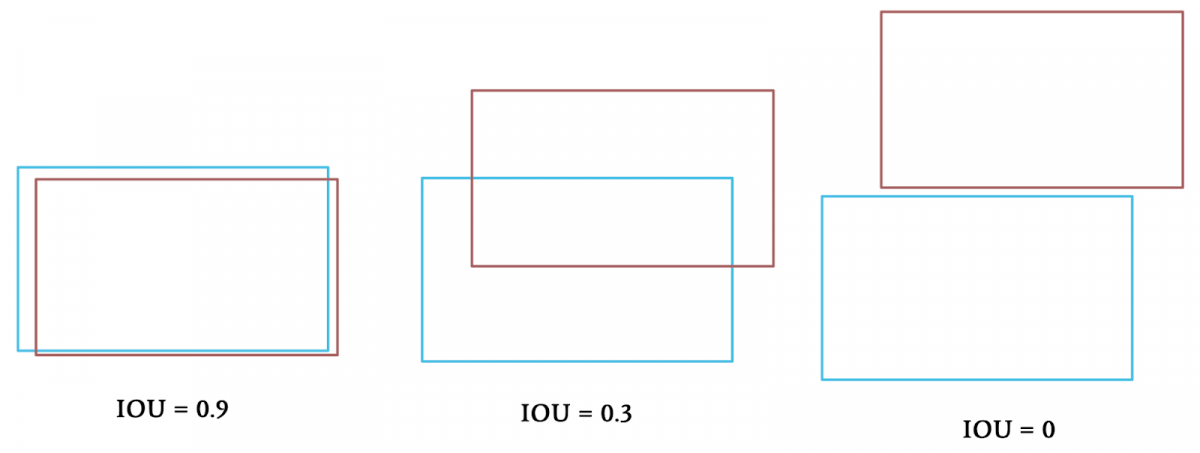
Although IOU is great, notice how it changes our problem from minimization (find the smallest euclidean distance) to maximization (find the biggest overlap).
Convolutional Cost (Visual Similarity / Dissimilarity)
Going deeper than calculating the overlap, we could also "look" inside the boxes using CNNs. This is what the DeepSORT algorithm uses, and although computationally expensive, this can help with overlaps.
To implement this, we use a Siamese Network, calculating the distances between two patchs of images. Here, we can use minimization (how dissimilar are they?) or maximization (how similar are they?).
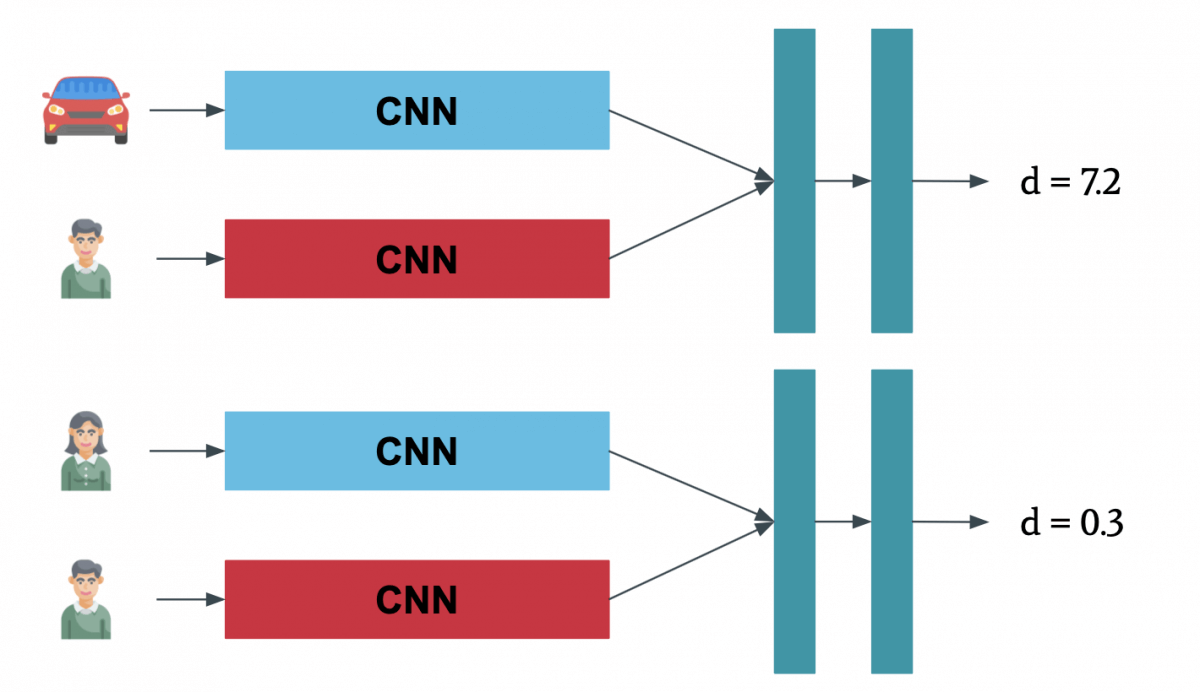
Here's an example of Deep SORT running:
A custom Cost Function
Depending on the problem, you want to be inventive with the matrix you have, and any suggestion that makes sense can help. The goal is to fill a matrix with costs. What's something else you could do? Combine all costs into a total cost variable. For example, combining IOU with Convolutional Costs! Or add other costs based on the bounding box shape together. You could add the class of the elements to the costs. In other words:
For any problem, you can design your own cost function, and convert that into a bipartite graph, and then use the Hungarian Algorithm on your problem.
How to deal with the "Edge Cases"?
We now know how to fill a cost matrix. But another problem remains to be solved: How do we deal with edge cases? What if I have an NxM matrix? Or what if I have a maximization problem and want to match the highest IOUs?
Let's take a look:
From NxN to NxM
Earlier, I also raised a question about what happens when we have 3 obstacles in image T, and 4 in image T+1? The trick here is conversion: take the maximum of the entire graph, and add a new column full of this value. If your maximum is 90, add a column full of 90.
Put differently, we want to add new edges to our graph. Let’s say we’re tracking obstacles and using IOU as a metric. On the detection side (time t), we have 4 obstacles. But on the tracking side (time t-1), we only had 3: a new detection has arrived.
Applying the conversion trick would give us the following:
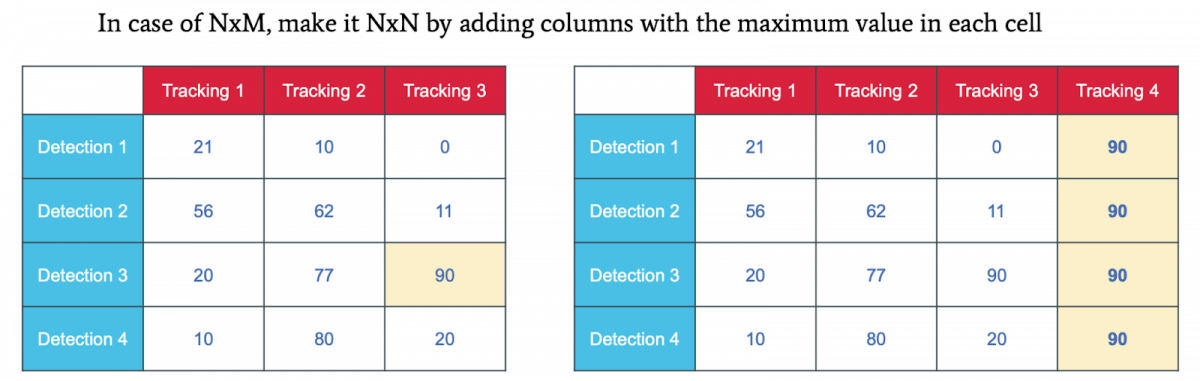
From Maximization to Minimization
The second problem is maximization. If you're having IOU values, you want the highest values to match. If you have similarity costs, you want the most similar examples to match. Here, the trick is to take the maximum value, and then remove the value of each cell to that maximum.
In the following example, the value of every cell on the left has been subtracted to 90, the maximum.
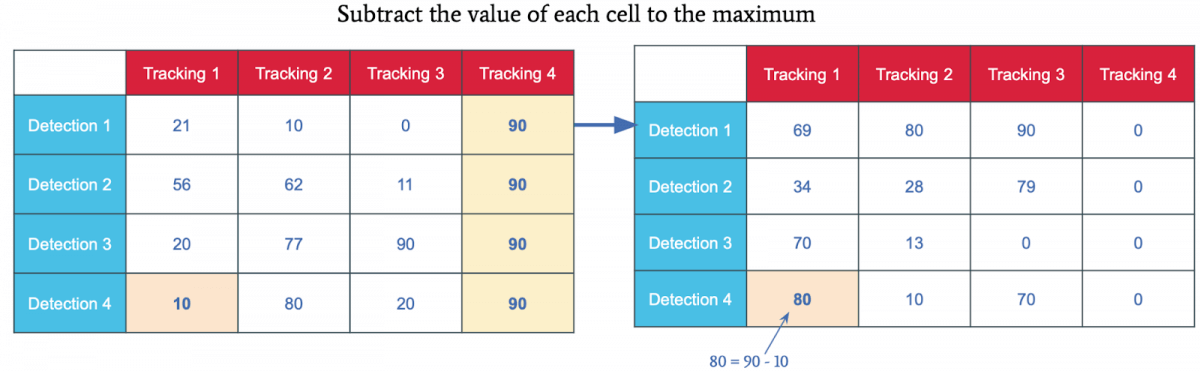
If you take the Detection 4, Tracking 1 — The cost is 10 —— the IOU is low. To convert that into a big distance, we’ll remove 10 from 90; and the value becomes 80. The big values such as 90 now become 0, and 0s become 90s. We can then run the optimization problem as before.
Summary & Conclusion
As you can see, the Hungarian Algorithm is a powerful optimization algorithm. We can use it to pair workers with jobs, but also to optimize advanced graphs.
It's an algorithm you should use often, but keep in mind how its complexity depends on your entries. If you have a graph with over 100 nodes, you'll need many more computations than if you have a smaller graph. It's sensitive to this.
Here's a short recap of what we've said, and takeaways from this article:
- The Hungarian Algorithm is helping you match two sets of elements linked by a cost metric. We call that an optimal assignment problem.
- When tracking bounding boxes, the cost can be the IOU, the Euclidean Distance, the Convolutional Cost, a shape cost, or even your own cost function.
- The algorithm works in 5 steps: we first reduce the matrix, then cross 0s, and finally reduce again until we can pair elements.
- If you have a maximization problem, such as IOU, you can always turn it into a minimization problem.
- If you have an NxM Matrix, you can make it an NxN matrix by adding columns with the maximum value.
- When coding in Python, you can call the function linear_assignment() from sklearn with a matrix to directly get the algorithm's output.
If you've been reading this far, it's very likely that you're interested in Bounding Box tracking or Sensor Fusion. I am teaching how to build these advanced applications of AI through my daily emails (which you should subscribe to). These emails are free, and I highly recommend you subscribe to them if it's not done yet.
I also have online courses. One of them goes through the entire implementation of the Hungarian Algorithm, as well as newer approaches such as the FairMOT algorithm. It's for engineers who want to go beyond simple object detection, and start making a direct impact in the field. It's called MASTER OBSTACLE TRACKING, you should check it out.