RADAR On Steroids: Why the Imaging RADAR is the future of self-driving cars
On June 13, 2021, during the finals of Roland Garros, Novak Djokovic was losing bad to its rival Stefanos Tsitsipas. After an hour of match, the world number #1 Â lost the first two sets 7-6, 6-2, and was now on a sure way to defeat. I was there, watching the game, and nobody else was feeling hope for Djokovic. He was done.
Then came the break, and something surprising happened... Just a few seconds after the referee declared "Set, Tsitsipas!", the serbian player went back to his chair, and then decided to... leave the court! Tsitsipas, as well as the entire crowd were left speechless. For minutes, everybody was wondering where the champion was; nobody had an answer. Was the game over?
And then, out of the blue, Djokovic came back. He was wearing a new outfit, but more than this, something had changed... The game continued, and Djokovic became extremely offensive, made almost no mistakes, and played so well he won the 3rd, 4th, and even 5th set, becoming the world champion of Roland Garros.
What happened during that break? Some people suspect Djokovic took drugs. Others suspect he simply did a mental mindset reboot. And some even declared Djokovic was on steroids all along, and he couldn't possibly have won that match.
Yet, he did, and after many tests, Djokovic was declared sober. So how did he win? During the break, something powerful happened to both Djokovic and Tsitsipas: change.
When something is not working as we expect, change is a powerful thing to do.
In the past few years, autonomous driving has started to look at RADARs as too noisy, too imprecise, and in one particular case, even degrading the output, having Tesla removing them from their self-driving cars.
This is why, recently, we've started to see the emergence of "imaging radars" — a new and different type of RADARs that some people even call "RADAR On Steroids".
So let's begin with the burning question:
What is an imaging RADAR?
Before this, let's go one level earlier and understand:
What is a RADAR? (The One Minute Intro to traditional RADARs)
In my Introduction to Radio Detection And Ranging, you can grasp some of the following ideas to understand what a RADAR is, and how it works:
- RADARs work by sending electromagnetic (EM) waves that reflect when they meet an obstacle
- Using the reflectivity, we can locate an object, and even classify it
- RADARs can work under all conditions, because EM waves do
- Most RADARs use the Doppler Effect to measure how fast objects are moving away or towards us (1D) — so it has direct velocity estimation without having to measure the difference between 2 frames
- Depending on the geometry and material of the object we're measuring, the measurement can work better or less. This means some objects could be invisible to RADARs, and this is even used in military.
- RADAR sensors are usually noisy, which make them an additional sensor, but never the main or only when in autonomous driving applications.
So if there are 2 ideas we can grasp, it's that RADARs are robust, but have poor resolution. Here is a video to express this idea:
The main problem being the resolution, we're going to run a mutation on the RADAR, and turn it into an Imaging RADAR. So let's see how.
Imaging RADARs
Although we'll have plenty of time to dive in the "how" of the imaging RADAR, I'd like to start by showing you the what it produces.
See? The resolution is simply incredible compared to the RADAR.
In this video, provided by startup bitsensing I had the pleasure to discover at CES 2023, we can see how an Imaging RADAR system produces point clouds, and have a resolution that can make them work much more as a standalone sensor.
This 4D RADAR from bitsensing can:
- Distinguish Heights: while usual RADARs work in 2D, this one has the third dimenson as well, with a FOV (Field of View) of 60°.
- Detect long range objects: up to 300 meters, which is quite good when typical RADARs do 100-150 meters. It can do that with small objects too.
- See precisely: The noise is removed, and we can see with an angular resolution of 1.5° (which means it can detect really close objects).
- Estimate Object Length: Which is due to the improvement in precision, height, range, and resolution.
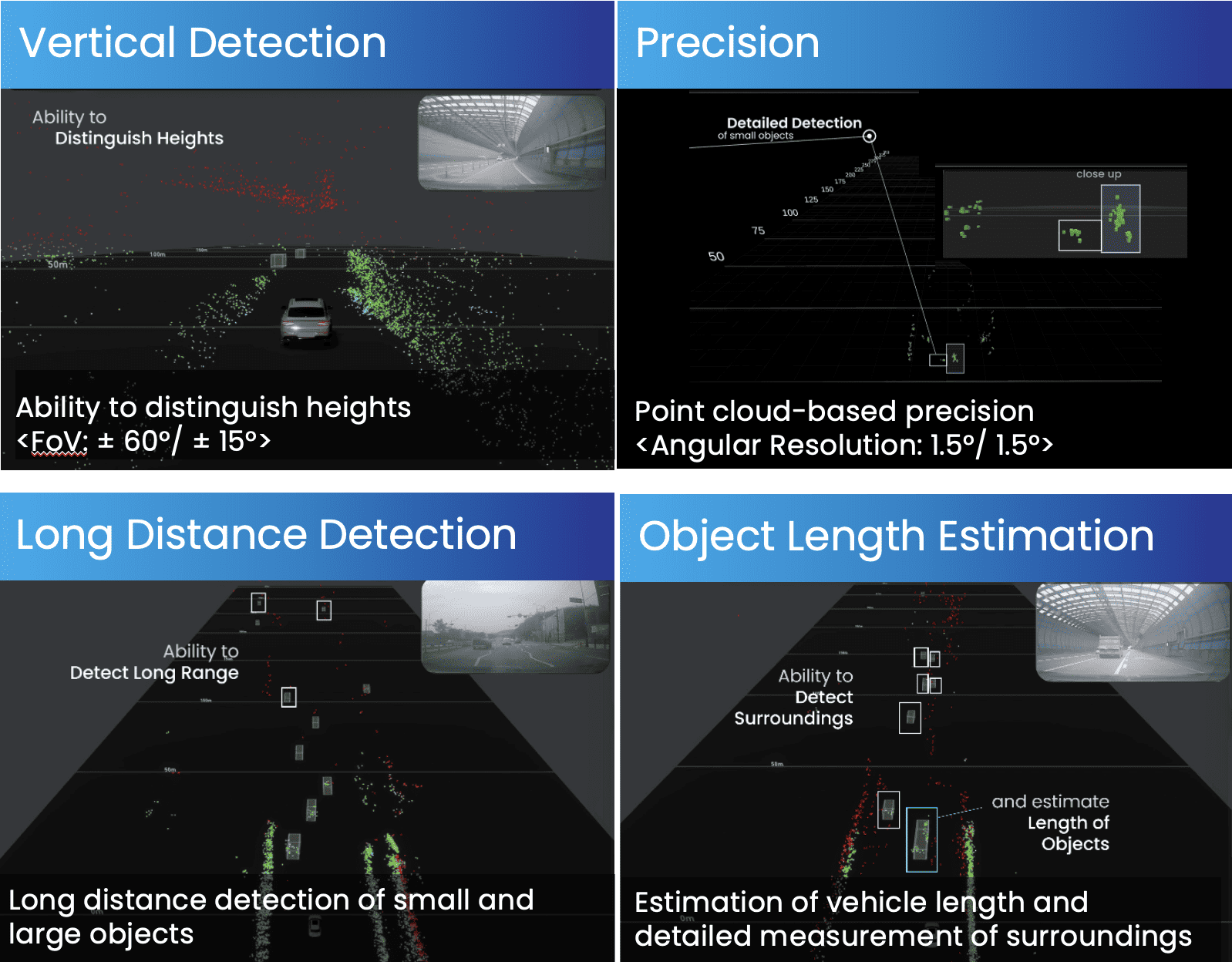
It's cool to note that while traditional RADARs detect X, Y, V, without the Z dimension, LiDARs detect X, Y, Z, but not velocity. Here, Imaging RADARs can detect X,Y,Z, and the Velocity.
So how does that work? So let's see how to get this result, and how imaging RADARs work.
How Imaging RADARs work
There are 3 things we're going to learn in this section, based on bitsensing sensor, they are:
- Multichip Cascading
- MIMO Antennas
- Signal Processing
And I know, you may not be familiar with this — me neither. So let's take it step by step to see how this works.
Multichip Cascading
To grasp the idea of multichip, you can simply imagine that most RADARs work with a single chip. This silicon MMIC (Monolithic microwave integrated circuit) chip can be connected to a limited radar antenna (ex. 3tx/4rx), which emits at a certain frequency, and does all the computations to find objects.
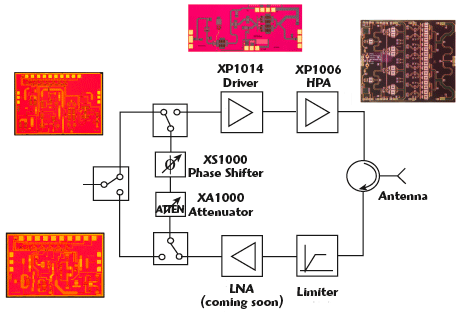
So what is multichip cascading? Simply the assembly of many of these chips. usually in a cascaded way, where the output of one chip/module shared the same LO (local oscillator) and performs synchronization.
This idea is usually implemented by high resolution cameras, but here we do it with RADARs, hence the word Imaging RADAR.
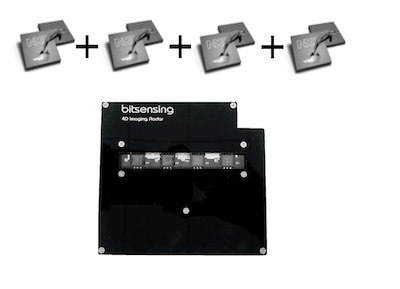
Which issue do you think this solves? Using multiple chips, we can orient them to see with more angle, than just a single chip. So we have an expanded field of view, but also a better resolution, and better robustness.
Now, if we have many chips, it means we have many antennas, so let's see this:
MIMO Antennas
The next idea is, I think, common to all Imaging RADARs: MIMO (Multiple Input Multiple Output) antennas.
MIMO antennas exploit spatial diversity by simultaneously transmitting and receiving multiple data streams over different antenna paths. They increase receiver signal-capturing power by enabling antennas to combine data streams arriving from different paths and at different times
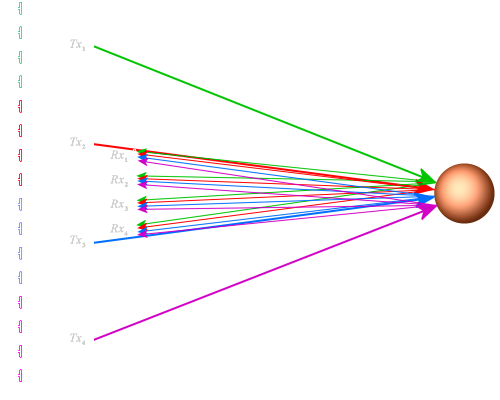
Traditional radar also uses MIMO, but because the imaging radar uses a lot more antennas, it's a lot more tricky to receive synchronized signals in a specific direction.
To allow this, the technology need a secret sauce...
- With traditional radars, we use MIMO through 3tx X 4rx (Tx= transmit | Rx: received) — so this is 12 channels.
- But with Imaging RADARs, we have 4 chip cascading, so we can generate 192 channels. With 192 channels, the performance and resolutions is way more advanced than traditional radars.
- The difficulty here is synchronizing these 192 channels. bitsensing uses a unique antenna layer patterning to synchronize the 192 channels and deliver high performance and resolution.
Finally:
Signal Processing
We so far understand that some Imaging RADARs use the multichip cascading system, as well as MIMO antennas, to increase spatial resolution, field of view, and get better performance.
But something to note is also how it processes signal. We now have 3D, and we also have the 1D Velocity reported by the Doppler effect. So RADAR signals can work in 3+1D. Thanks to these, we can produce a RADAR image, or a point cloud.
As a reminder, the Doppler effect tells how an object is moving away or towards you (in 1 dimension) — this is what FMCW LiDARs stole to the RADAR to become 4D.
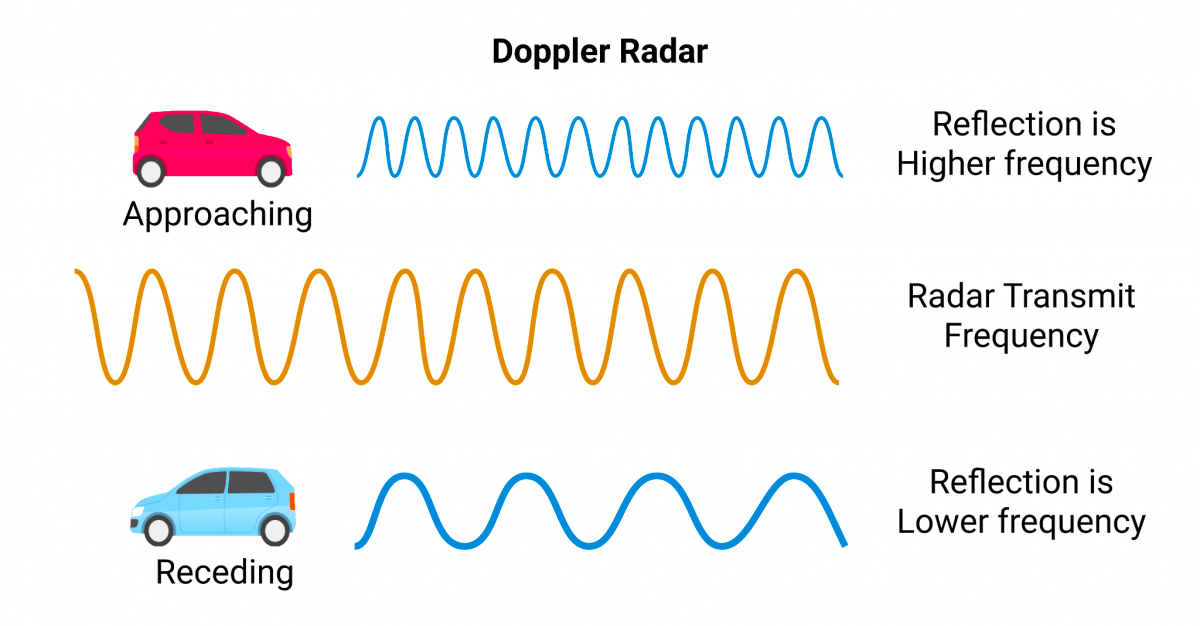
There are other things you should note, such as the idea of RADAR Cross Section, or the idea of RADAR backscatter (waves bouncing back to the RADAR) which are explained in my RADAR article. And thanks to this, we can come up with an Imaging RADAR.
Let's now do a brief overview of the field:
The different types of RADARs and Imaging RADARs
Just like there are several types of LiDARs, there are several types of RADARs. And because of this, there are several types of Imaging RADARs. Let's begin with a quick overview of RADARs:
The overview and different types of RADAR systems
RADARs are 100 years old sensors, so you can imaging we built several types of them. I am no RADAR expert, I never even really "worked" on RADARs, besides some experimentation projects, so I'm probably unfit to give you an overview. But on the entire internet, nobody else will, so let me try a simple mindmap (and please correct me if you see issues):
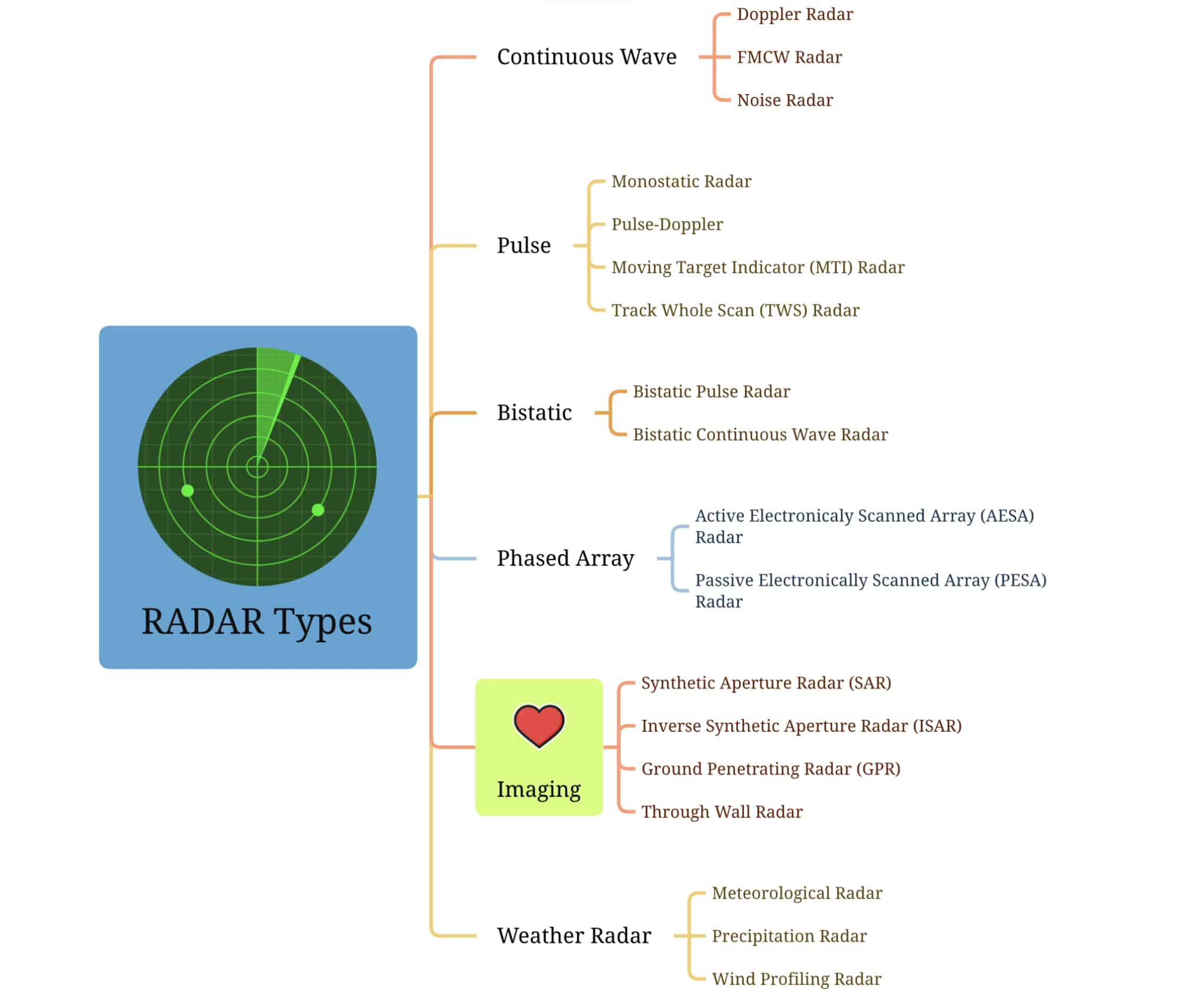
Notice how we have several types, such as:
- Continuous Wave: In CW radar, the transmitter continuously emits a sinusoidal waveform at a specific frequency. The receiver picks up the reflected signal, which contains information about the range, velocity, and other characteristics of the detected objects.
- Pulse: In pulse RADARs, the transmitter emits brief pulses of electromagnetic energy, which travel through space and interact with objects in the environment. The receiver then listens for the echoes or reflections of these pulses that bounce back from the targets.
- Bistatic: In a bistatic RADARs, the transmitter emits the radar signal towards the target, and the receiver detects the echoes or reflections of the signal after it interacts with the target. The transmitter and receiver can be stationary or in motion, and they can be located on different platforms, such as aircraft, ships, or ground-based stations.
- Phased Array: In a phased array radar system, each antenna element can be individually controlled in terms of phase and amplitude. By adjusting the phase and amplitude of the signals across the array, the radar system can steer and shape the radar beam electronically, without the need for physically moving the antenna.
While the military and defense world usually uses phased array RADARs, the self-driving car industry, to my knowledge, mainly uses Continuous Wave RADARs. Mainly because this type of RADAR is the best for the Doppler effect.
Now if we zoom in the last type:
Zooming in the Imaging RADAR
You may have noticed I talked about a few types. So let's see them briefly:
- Synthetic Aperture Radar (SAR)
- Inverse Synthetic Aperture Radar (ISAR)
- Ground Penetrating Radar (GPR)
- Through Wall Radar
All of these have their own specificities — some are used mainly in aircrafts, and others are more suited for mapping. I believe the self-driving car field will use Synthetic Aperture Radars when they're mature. Today, SLAM is still the trend.
Wait! Aren't FMCW LiDARs better?
Recently, we saw another sensor evolve to the 4th dimension: LiDARs. LiDARs (Light Detection And Ranging) moved from being a 3D sensor, to being a 4D sensor, and this mainly by taking the FMCW ability of the RADAR, and thus detecting radial velocity.
Are they better? They can be, and running a side-by-side comparison between the best 4D LiDAR and the best 4D RADAR would make a lot of sense (if you're a company reading this, just ship it, and I'll do the comparison!).
I wouldn't say one is necessarily better than the other; and both have one major flaw: they only detect the speed in one dimension. Â This means that they know whether an object is approaching or receeding, but not how fast it moves laterally, at least not directly.
An example with Aeva's FMCW LiDAR, notice how cars are either red or blue, but never green, yellow, etc...
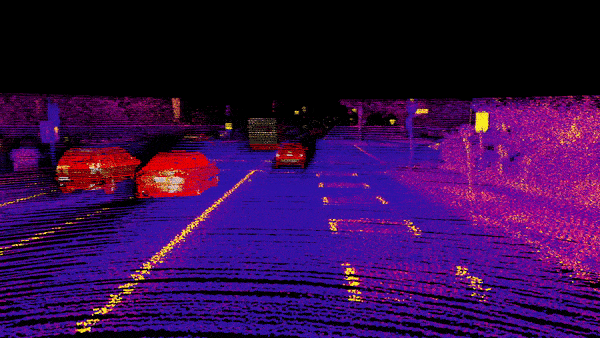
If you want to learn more about FMCW LiDARs, I have an entire article here, and a quick comparison of the strength of the two below:
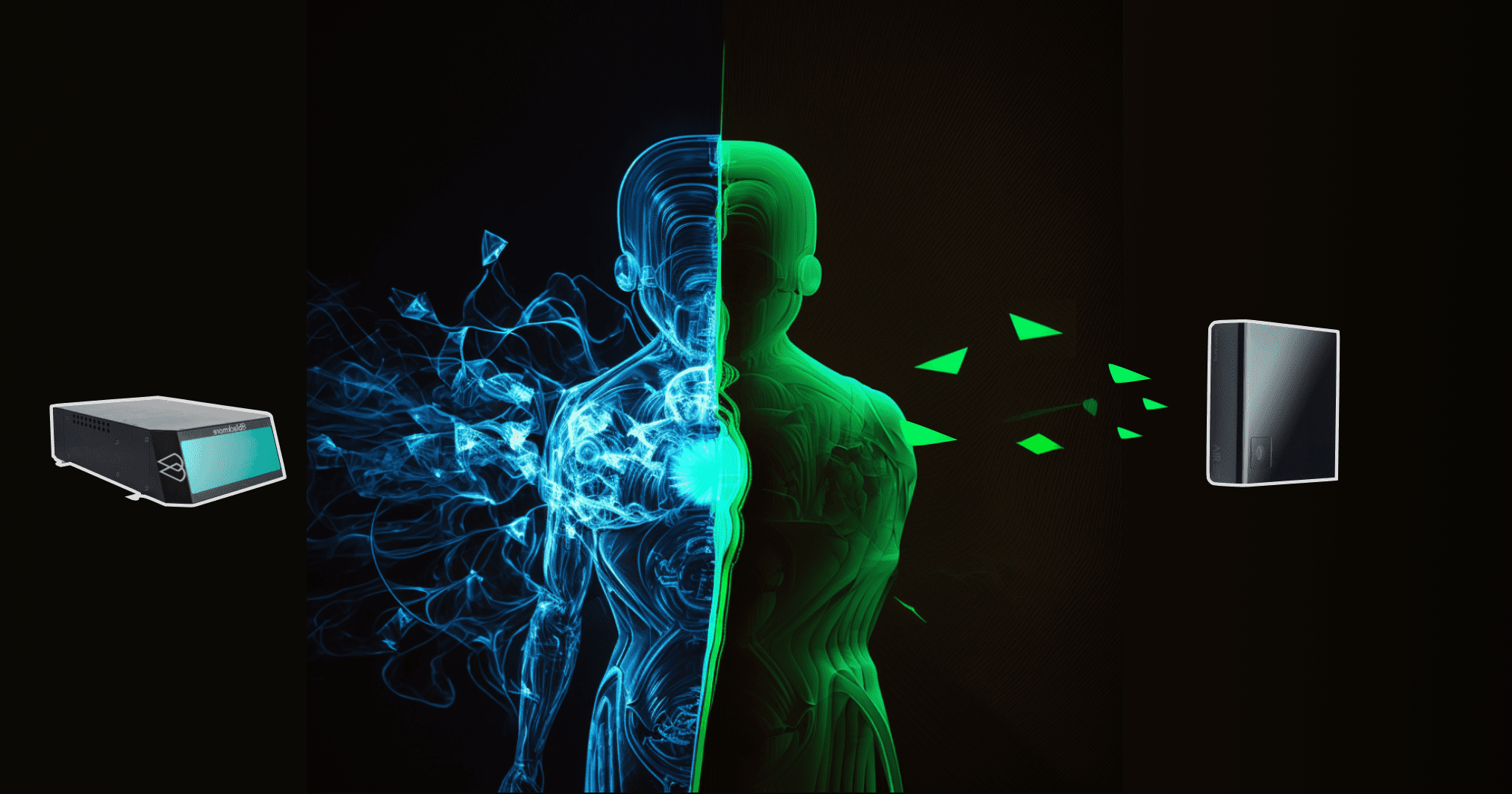
An example of 4D RADAR: bitsensing
Earlier, I showed you how bitsensing, a startup who raised 11M$ in Late 2022, is building an Imaging RADAR. A few months ago, they sent me a presentation of their revolutionary sensor, and showed how they could do SLAM, Object Detection, and even freespace detection using this sensor alone.
Recently, I met them in Las Vegas, and through their demo, I could record one of their key feature: precision.
As we showed before, precision is done thanks to the MIMO system, and I believe partly thanks to the multichip cascading system as well. There are probably a lot of good operations they are going to implement next.
When is their time to market? bitsensing is preparing mass production by 2025 with a top tier OEM.
For the entire Imaging RADAR industry, it's all still blurry. The sensor seems quite new, and the difficulty to switch from one type of sensor to another is strong in self-driving cars.
On that note, let's do a quick summary of what we learned in this article:
Summary
- RADARs have been in automotive, military, aircrafts, and many other industries for a hundred years. They use EM waves to measure range and velocities directly, but are low resolution.
- Imaging RADARs improve 4 main elements compared to RADARs: vertical detection (3D), precision, range/long-distance, and object length measurement.
- Imaging RADARs use multichip cascading and MIMO antennas to improve the field of view, resolution, and precision.
- They also add signal processing to these 2 to allow for vertical detection.
- Multichip cascading is the idea that we can combine several silicon chips, similarly to how high resolution cameras do, and therefore have this imaging capability.
- MIMO antennas combine multiple input signals to provide a wider field of view, better resolution, similarly to using multiple speakers in a concert show.
- There are several types of RADARs, such as Pulse RADAR, Continuous Wave, Phased-Array, etc... and the use of them depends on the use case you have. Similarly, there are several types of Imaging RADARs.
- Companies like bitsensing provide Imaging RADARs, and are currently actively integrating the self-driving car industry.
Next Steps
If you liked this article on Imaging RADARs, you may like other articles I have for you, on FMCW LiDARs, or this comparison of Imaging RADARs vs FMCW LiDARs.
If you want to get daily training on autonomous systems, Deep Learning, and Sensor Fusion, I'm sending an email per day to a list of 10,000+ engineers. These emails are private, written freshly every morning, and never published anywhere else.
--> Subscribe here and join 10,000+ Engineers!